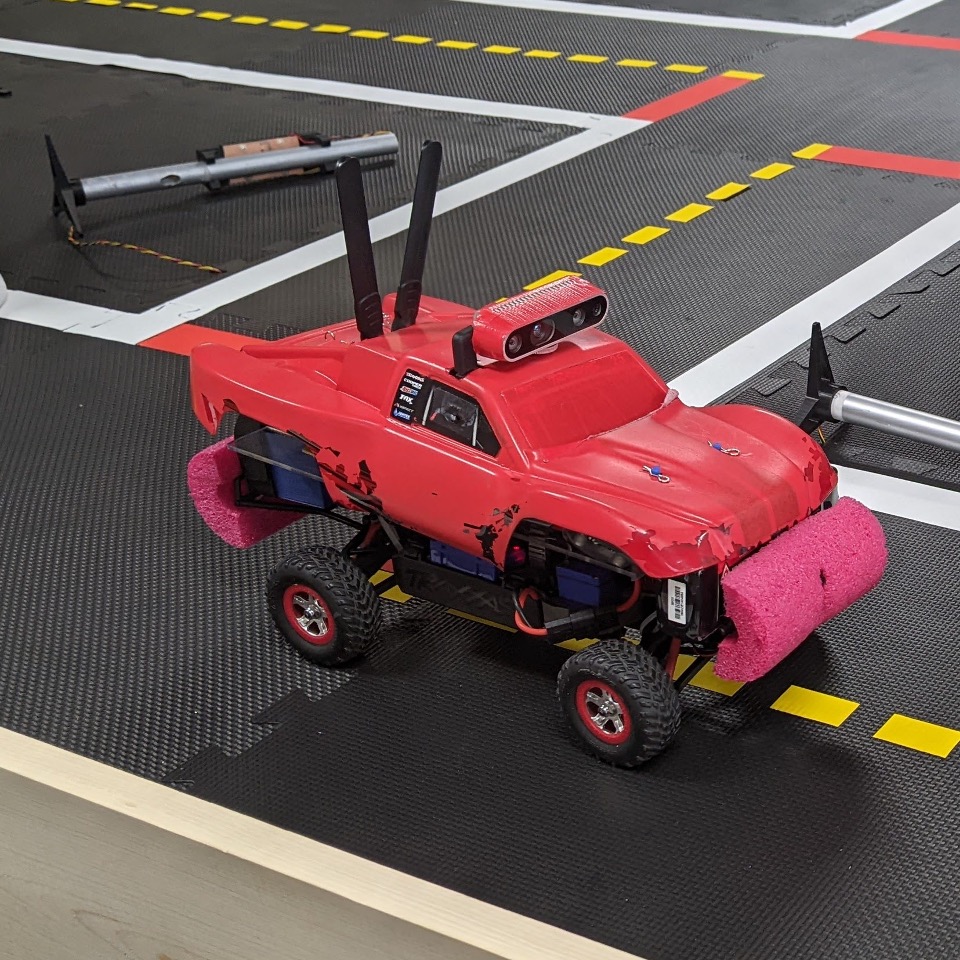
As part of my robotic vision coursework, I coded an autonomous monster truck that could navigate indoor hallways at high speeds. Using an NVIDIA Jetson TX2 board and Intel RealSense camera, I implemented computer vision algorithms that allowed the vehicle to detect and stay within hallway boundaries, smoothly navigate corners, and race toward the finish line. This hands-on project brought together advanced concepts in color image processing and path planning, demonstrating how computer vision enables real-world autonomous navigation.
Project Objectives
- Navigate autonomously through indoor hallways
- Handle corner navigation
- Reach the finish line as quickly as possible
Hardware Setup
The project is built around a modified Traxxas Slash 4×4 RC car, enhanced with sophisticated computing and sensing capabilities. Here’s what powers our autonomous system:
Core Components
- Traxxas Slash 4×4 RC car (base vehicle)
- Intel® RealSense™ Depth Camera D435i
- NVIDIA Jetson TX2
- Custom interface board with Arduino Nano
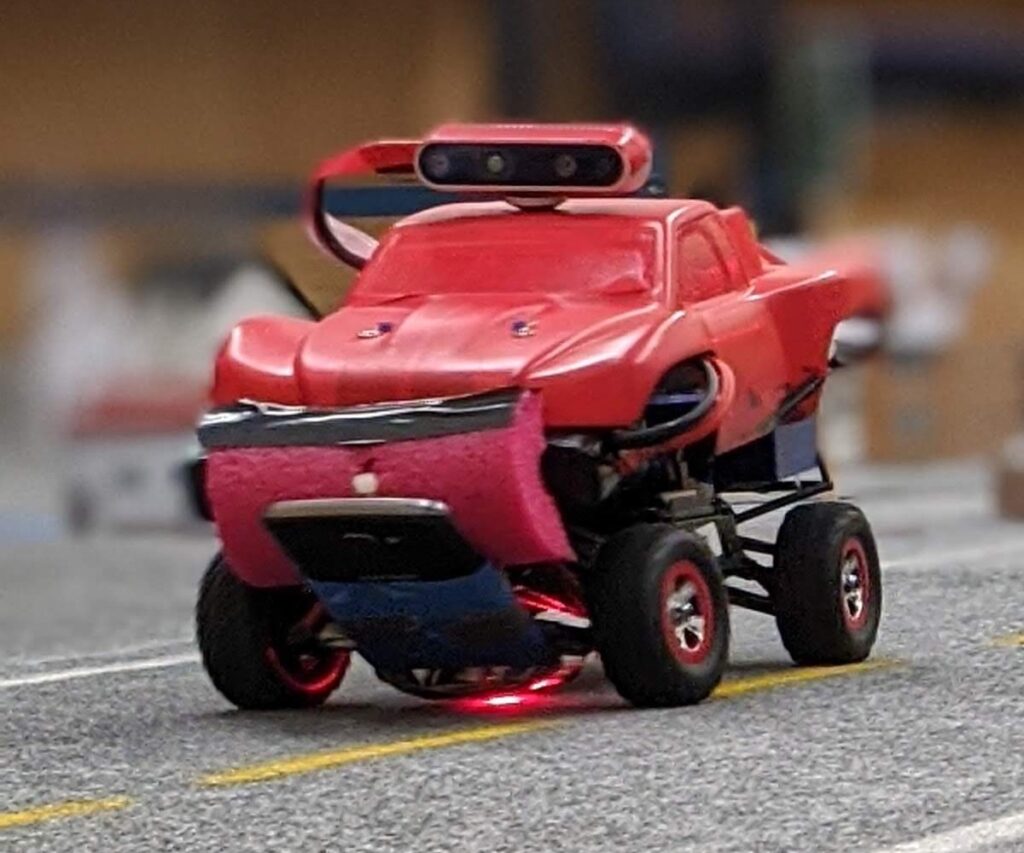
Technical Implementation
The autonomous navigation system was implemented using Python and OpenCV, with a state machine architecture to handle different driving scenarios. The system processes two main inputs:
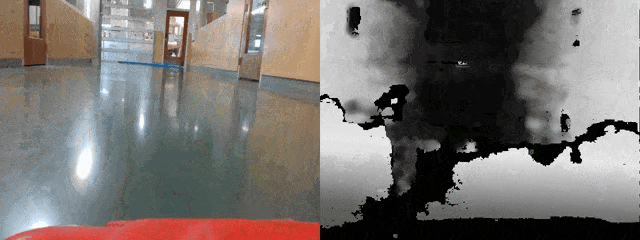
- RGB Camera Feed:
- Used for detecting blue pool noodles that mark turning points
- Implements color thresholding and morphological operations
- Triggered turn state based on detected noodle area
- Depth Camera Feed:
- Divides the view into a 11 vertical regions for depth analysis (seen below)
- Identifies the furthest navigable region
- Uses a cubic function to map the furthest navigable region to steering commands
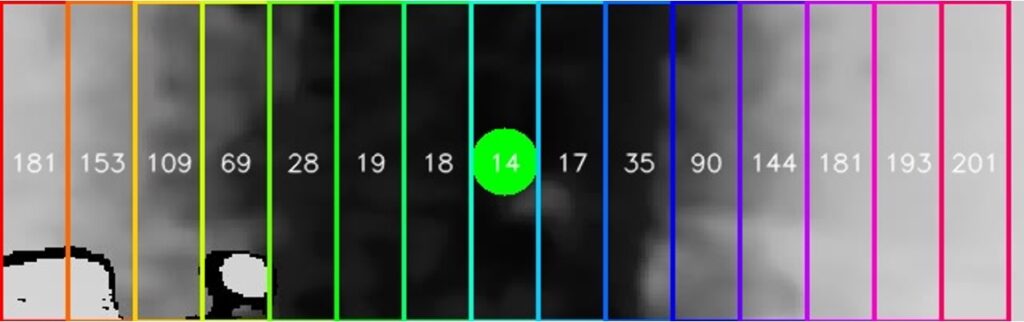